Welcome to Nural's newsletter where you will find a compilation of articles, news and cool companies, all focusing on how AI is being used to tackle global grand challenges.
Our aim is to make sure that you are always up to date with the most important developments in this fast-moving field.
We now have Jobs section currently featuring an exciting data scientist role at startup AxionRay
Reach out to advertise your own tech roles!
Packed inside we have
- DeepMind collaborates with chemists using machine learning to better predict the distribution of electrons
- IBM collaborates with chemists using machine learning to develop new molecules and materials more quickly
- and Eleuther launches the largest open source AI language model
If you would like to support our continued work from £1 then click here!
Graham Lane & Marcel Hedman
Key Recent Developments
DeepMind collaboration tames quantum complexity

What: The properties and interactions of atoms, molecules and materials can be predicted by understanding the behaviours of their electrons. The distribution of electrons is subject to universal laws but the interactions are immensely complicated and not fully understood. Density Functional Theory (DFT) is a technique to calculate approximately where electrons will go and, by extension, how atoms and molecules surrounded by electrons will act. Researchers from DeepMind have applied a machine learning approach to this complex problem. Rather than calculating from first principles, a model is trained on known examples and this is used to predict the distribution in unfamiliar molecules. The model outperforms existing benchmarks but there are limitations, particularly that the training data is only available for some parts of the periodic table.
Key Takeaways: The research demonstrates the success of combining DFT with modern machine-learning methodology. The machine learning is not a model to replace existing work but a tool to help researchers.
Paper: Pushing the frontiers of density functionals by solving the fractional electron problem (subscription required)
IBM accelerating molecular optimization with AI
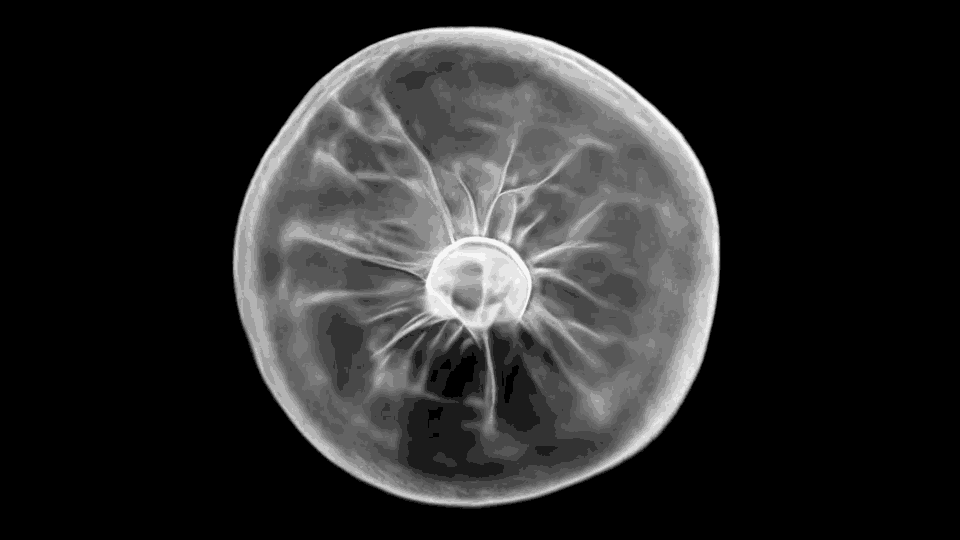
What: Addressing grand challenges demands new molecules and materials, from antimicrobial and antiviral drugs to more sustainable photosensitive coatings and next-generation polymers to capture carbon dioxide at source. Starting from a known molecule gives a head start in design and production. The problem is that tweaking a molecule can produce an unmanageable number of variants. IBM is addressing this problem by using AI to find the best candidate variants for further research. The researchers used this approach in the case of Covid-19 to investigate candidate drugs that maintained their effectiveness whilst improving their binding affinity.
Key Takeaway: This is an example of using AI as a tool to assist practical research. The researchers propose that the overall methodology, which they call Query-based Molecular Optimization, may also be applicable in accelerating other areas of research.
A new, open source, publicly accessible AI language model

What: Eleuther.ai, a grassroots collective of researchers working to open source AI research, have launched what they claim is the largest publicly accessible pretrained general-purpose AI language model, called GPT-NeoX-20B. The model has 20 billion parameters and was trained on EleutherAI’s curated collection of datasets. The model is accessible through a fully managed API. The initiative is motivated by “the belief that open access [to AI large language models] is critical to advancing research in a wide range of areas” including AI safety, interpretability and sustainable scalability.
Key Takeaway: The release of yet another AI Large Language Model may not address a grand challenge in its own right. However, the release of a publicly accessible model is an important step supporting scientific progress and knowledge sharing. It seeks to counter-balance the concentration of power in the hands of Big Tech companies operating closed and proprietary systems.
Paper: GPT-NeoX-20B: An Open-Source Autoregressive Language Model
AI Ethics
🚀 Algorithmic impact assessment: a case study in healthcare
The report claims to be the first detailed proposal for an algorithmic impact assessment for data access in a healthcare context, focusing on the UK National Health Service.
🚀 Jury Learning: Integrating Dissenting Voices into Machine Learning Models
An interesting approach to fairness in machine learning focusing on the role of the humans who label the underlying data set. For example, in assessing online toxicity, the data labelled by groups who may be targets of toxicity (such as women and black people) might carry extra weight.
🚀 Chinese scientists create AI nanny to look after embryos in artificial womb
Are we living in the Metaverse, or a Simulation?
Other interesting reads
🚀 Andrew Ng: The AI pioneer says it’s time for smart-sized, “data-centric” solutions to big issues
An enlightening interview with Andrew Ng, covering foundation models for computer vision, data-centric AI, the shift from “big data to good data”, the problems of labelling data and how this can be linked to bias in data.
🚀 Emerging Trends: SOTA-Chasing
AI researchers will proudly announce that their latest model exceeds the current State of the Art (SOTA). A new book from Cambridge University Press discusses the numerous ways in which this constant pursuit of SOTA can be dysfunctional.
🚀 M2D2: Molecular Modeling And Drug Discovery
M2D2 is a new website dedicated to molecular modelling and drug discovery. There is a also a series of weekly talks ranging from applied research papers to open source projects. The organisers hope to “demystify AI for drug discovery and make the field more accessible for newcomers”.
🚀 New DOD Chief Digital Artificial Intelligence Office Launches
The weaponisation of AI remains a persistent concern. The U.S.A. Department of Defense is now seeking a chief digital and artificial intelligence officer to “preserve its military advantage”.
Jobs
Data scientist - AxionRay
Axion are looking to hire a talented NLP DS lead as they enter hypergrowth. Axion is a stealth AI decision intelligence platform start-up working with electric vehicle engineering leaders to accelerate development, funded by top VCs.
Comp: $100k – $180k, meaningful equity!
If interested contact: marcel.hedman@axionray.com
Cool companies found this week
ML deployment
Wallaroo - addresses the “last-mile” problem of deploying ML models efficiently into production. The company has won $25 million in round A funding from Microsoft’s M12.
ML data quality
Superconductive - the company behind the open-source tool for data quality called Great Expectations has raised $40 million in round B funding.
AI-powered dubbing
Deepdub - provides AI-powered dubbing services for film, TV, gaming, and advertising that splits and isolates voices and replaces them in the original tracks. The company has raised $20 million in round A funding.
And Finally, if you don't like flying cockroaches, look away now ...
AI/ML must knows
Foundation Models - any model trained on broad data at scale that can be fine-tuned to a wide range of downstream tasks. Examples include BERT and GPT-3. (See also Transfer Learning)
Few shot learning - Supervised learning using only a small dataset to master the task.
Transfer Learning - Reusing parts or all of a model designed for one task on a new task with the aim of reducing training time and improving performance.
Generative adversarial network - Generative models that create new data instances that resemble your training data. They can be used to generate fake images.
Deep Learning - Deep learning is a form of machine learning based on artificial neural networks.
Best,
Marcel Hedman
Nural Research Founder
www.nural.cc
If this has been interesting, share it with a friend who will find it equally valuable. If you are not already a subscriber, then subscribe here.
If you are enjoying this content and would like to support the work financially then you can amend your plan here from £1/month!