Welcome to Nural's newsletter where you will find a compilation of articles, news and cool companies, all focusing on how AI is being used to tackle global grand challenges.
Our aim is to make sure that you are always up to date with the most important developments in this fast-moving field.
Packed inside we have
- Nvidia upgrades its digital twin platform
- A socio-technical approach to AI bias
- and the shortcomings of "explainable AI"
If you would like to support our continued work from £1 then click here!
Graham Lane & Marcel Hedman
Key Recent Developments
Nvidia’s digital twin platform will change how scientists and engineers think

What: Nvidia has announced several significant upgrades to its scientific computing for digital twins platform and released these capabilities for widespread use. This includes the release of a “physics-informed AI tool”. Rather than encoding the laws of physics for use in simulators, this approach enables the creation of AI-based surrogate models that abstract physics principles from observed real-world data. Another enhancement supports the training of neural networks that reflect 3D spatial states. Nvidia also announced a new range of processors optimised for AI workloads and hinted at a ‘world’s fastest AI supercomputer’.
Key Takeaways: The use of digital twins continues to develop in both climate change work and in manufacturing processes. Accurate physics simulation is important in a number of areas such as environmentally-friendly building design. Digital simulation environments are used increasingly to train AI models for robots which are then transferred, fully operational, to the physical robot. An example is the simulation-trained robotic cheetah which can run very fast but uses a peculiar and unique AI-designed gait.
There’s more to AI bias than biased data, NIST report highlights
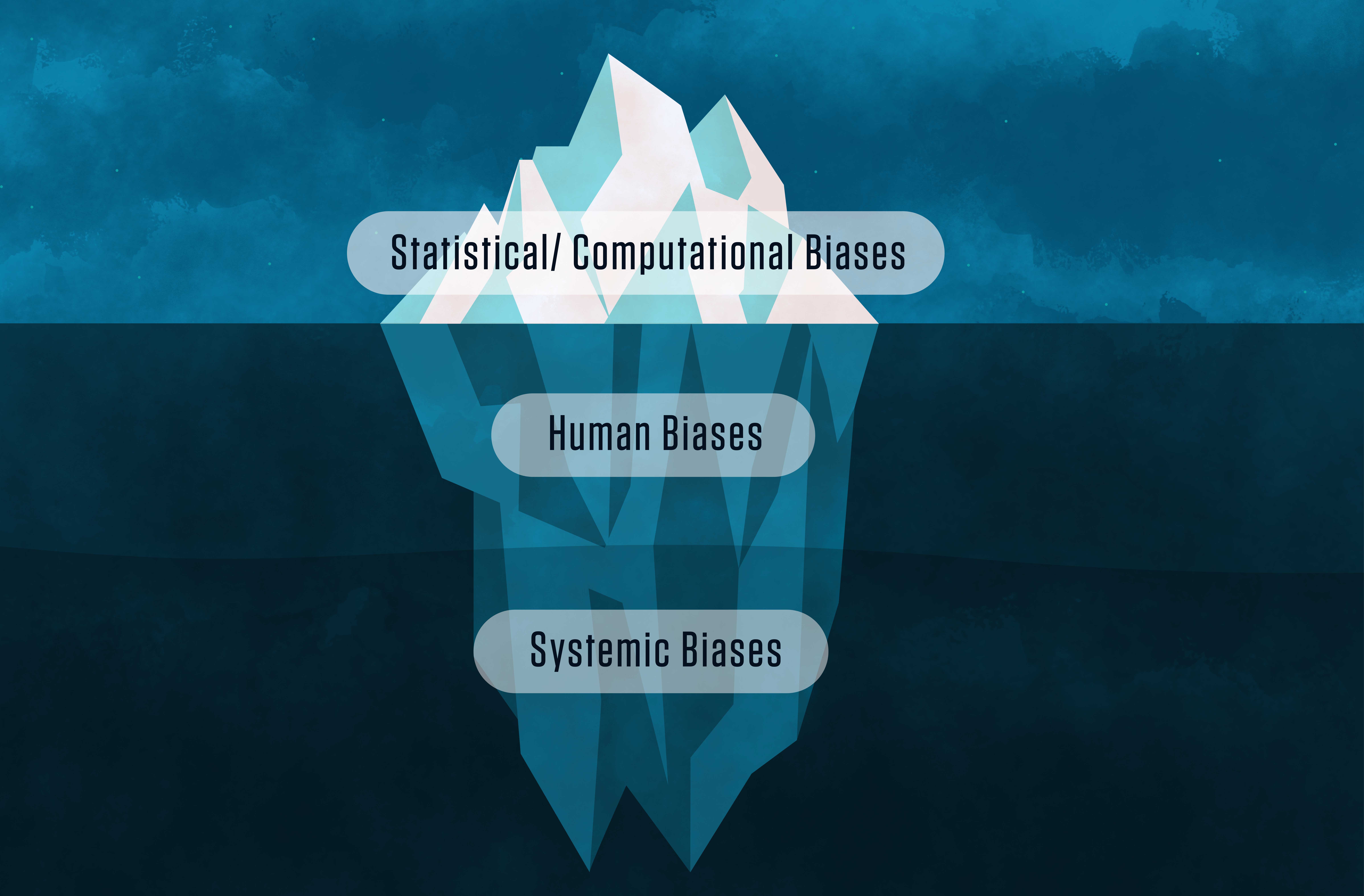
What: The influential U.S. National Institute for Standards and Technology (NIST) issued a milestone report about identifying and managing bias that frames the problem and provides practical advice. NIST is hugely influential and the report has been called “America’s AI bias gold standard”. The report argues that current attempts at mitigation of bias are focused on computational factors such as the representativeness of datasets and fairness of algorithms. However, “human and systemic institutional and societal factors are significant sources of AI bias as well”. These are currently overlooked and need to be addressed through a socio-technical perspective. One of the interesting practical recommendations is “aligning pay and promotion incentives across teams to AI risk mitigation efforts, such that participants in the risk mitigation mechanisms… are truly motivated to use sound development approaches, test rigorously and audit thoroughly.”
Key Takeaway: There is a growing move towards a broader social-economic perspective in AI ethics. See also the AI Decolonial Manyfesto (sic) in this newsletter.
What’s wrong with “explainable AI”

What: This is an insightful critique of the short-comings of current “explainable AI” in a medical context. Explainable AI has been added on to, for example, existing medical imaging systems in order to explain predictions. But practitioners complain that these explanations themselves don’t make any sense. For example, a heat map showing the elements most heavily weighted by the algorithm when predicting a lung image simply highlighted a large quadrant of the image with no further explanation, leaving human practitioners none the wiser. Furthermore, different methods of explainable AI have been demonstrated to provide different explanations for the same image.
Key Takeaway: Somewhat counter-intuitively, the conclusion is that rather than focusing on explanations, doctors should really be concentrating on performance and whether the model has been tested in a rigorous, scientific manner. Medicine is full of drugs and techniques that doctors use because they work, even though no one knows why. In other words, for AI in medicine, the key is validation, not explanation.
AI Ethics
🚀 The movement to decolonize AI: centering dignity over dependency
In the same week as the NIST report (above), this opinion piece takes a socio-economic perspective on AI ethics: “there’s a tendency among prominent AI practitioners … to think we can fix … harms by getting better data… but even if a large, very precise dataset is available, that dataset is a digital representation of society as it is: The producers of the data have biases that are difficult to remove from the data, as do those who make decisions regarding what data to collect, how to collect it, and what to use it for. So having more data doesn’t solve anything”.
It promotes the AI Decolonial Manyfesto (sic)
🚀 Machine learning experts - Margaret Mitchell
Meg Mitchell talks about AI ethics and the use of “Model Cards” to address bias in AI models.
🚀 Why AI ethics are important:
As an experiment, an AI model generating medicines was tweaked to produce toxins and suggested 40,000 possible chemical weapons in six hour.
The world’s largest website, TikTok, reportedly fed war disinformation to new users within minutes of joining the system, even if they didn’t search for Ukraine-related content.
Other interesting reads
🚀 Tackling climate change with machine learning
This ACM Computing Survey provides a detailed study of where ML can be applied with high impact in the fight against climate change, through either effective engineering or innovative research. It includes contributions from a range of leading academics and practitioners including Andrew Ng (Stanford), Demis Hassabis (DeepMind) and Joshua Bengio (Montreal Institute for Learning Algorithms).
🚀 Scientists develop ‘holy grail’ method to identify the ageing mosquitos which cause malaria
Shining infrared light on mosquitos provides information that can be analysed by AI image recognition to identify those mosquitos that may be carrying malaria.
🚀 AI and robotics uncover hidden signatures of Parkinson’s disease
The discovery of novel disease signatures has value for diagnostics and drug discovery, even revealing new distinctions between patients.
Cool companies found this week
System on a Chip
Quardric - have designed a unique “System on a Chip” first starting with the algorithms, compiler and software, and only then building the required architecture. This has applications in edge computing such as automated driving but can also be used to apply safety solutions to traditional automotive applications. The company has raised $21 million in round B funding.
AI model quality
TruEra - provides a software suite to help ensure AI model quality providing performance analytics in the development phase, and then monitoring in live use to ensure rapid debugging and optimal ongoing performance. The company has secured $25 million in round B funding.
ML abstraction
Run:AI - in normal IT applications there has been a strong move to abstraction between the software layer and the hardware layer through technologies such as containerisation and virtualisation. The same is not true of machine learning applications, which often exhibit dependencies between software in the form of ML models and specialized hardware. This company offers an ML hardware virtualisation solution and has attracted $75 million in round C funding
And finally ...
AI/ML must knows
Foundation Models - any model trained on broad data at scale that can be fine-tuned to a wide range of downstream tasks. Examples include BERT and GPT-3. (See also Transfer Learning)
Few shot learning - Supervised learning using only a small dataset to master the task.
Transfer Learning - Reusing parts or all of a model designed for one task on a new task with the aim of reducing training time and improving performance.
Generative adversarial network - Generative models that create new data instances that resemble your training data. They can be used to generate fake images.
Deep Learning - Deep learning is a form of machine learning based on artificial neural networks.
Best,
Marcel Hedman
Nural Research Founder
www.nural.cc
If this has been interesting, share it with a friend who will find it equally valuable. If you are not already a subscriber, then subscribe here.
If you are enjoying this content and would like to support the work financially then you can amend your plan here from £1/month!