It’s no secret that human driven climate change is altering the delicate balance of the world as we know it. The evidence is abundant whether that be the incresing number of scorching wildfires or the onset of destructive tropical storms, global warming is and will continue to disrupt the world if no intervention occurs.
At stake are countless lives and vast amounts of money, as projections from the Natural Resources Defense Council (NRDC) suggest. According to the NRDC, the cost of overhauling energy systems towards greener sources, an undertaking that has long caused many business people to balk at costs, pales in comparison to the financial damages that will directly result from a changing climate. At risk communities include coastal areas because of rising sea levels and intense hurricanes resulting from glacier meltings and increasing water temperatures and agricultural areas due to increased drought. The NRDC predicts that the U.S. will spend almost 2 trillion dollars in relief for such plights in the year 2100, up almost 7 times from what it predicts the U.S. will spend in 2025 [1].
As these disasters become more common place, weather prediction will play a huge role in mitigating the adverse effects and saving millions of lives alongside billions of dollars. An area of growing interest is the role that deep learning can play in this field and this has been facilitated by the growing sophistication of computer graphic technology. This article explores the emerging evidence that deep learning can help in the weather prediction process.
Non-AI aside
Of course, the focus of the work at Nural is to explore how AI is being used to tackle global grand challenges, but it is important to realise that AI is merely a tool that must be implemented alongside other solutions as well as regulatory change. Investment must be made in renewable energy alternatives and negative carbon emission techniques such as BECCS.
20 companies worldwide can be directly linked to 35% of carbon emissions [3]. Thus, it does not simply fall on consumers to change their habits, but rather serious regulatory changes must be implemented to facilitate change.
What is the current state of weather prediction?
Unfortunately for AI enthusiasts, deep learning models are not currently considered the state of the art as far as weather prediction. The field is currently dominated by physics-based computer simulations, in which the governing equations of the atmosphere and ocean are solved on a discrete numerical grid, (yes, there are computer algorithms outside of machine learning!).
The incumbent method requires meticulous calculation of the physics of the atmosphere which has a large computational power requirement. On the other hand, deep learning contrasts this approach with a “data-driven” process which seeks to extrapolate more “efficient representations” of the underlying trends and thus drastically slashes the computational power required leading to energy and cost savings [2].
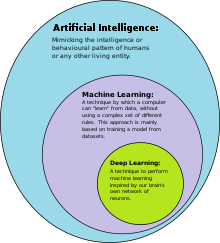
The opportunity
One study [4] compares deep learning models known as LSTM and TCN to state-of-the-art well established weather research and forecasting (WRF) models. They are also compared to traditional ML algorithms such as Random Forest and SVR.
The deep learning models are "lightweight" because they require far less computational power than the traditional WRF models and it is found that they are particularly advantageous in short term weather forecasting and even outperformed the WRF in the 12 hour range.
A further pragmatic advantage of using deep models over the WRF is that the WRF requires access to data that “requires a minimum of 3 h of access GPS data after taking the atmospheric measurements.” The drawback here in WRF models is that once they are shown the data, it is already at least 3 hours old, which can lead to more inaccuracies. Therefore, it becomes more practical to use “local weather station data,” which beckons the use of deep learning models because of their cheap computational cost and portability. A final interesting facet of the deep learning models in the study was that they actually accept less data than the WRF models, and in spite of this still outperformed the WRF in some time ranges.
The highlights
- Portability
- Reduced computational requirement
- Better predictions on shorter time frames
Emerging Research
Deep learning in this particular usecase shows a lot of promise but there are still some drawbacks such as the lack of consistent data sets for comparison. However, there are tools being developed to combat this.
One such tool that just made a splash in the development of deep learning models for climatology and meteorology is called WeatherBENCH, which addresses several weaknesses in the deep learning approach. Namely, deep learning models in the field “lack of a common dataset and evaluation metrics make inter-comparison between the proposed models difficult,” and “there is currently no standard approach for evaluating the proposed models” [2]. To combat these problems, WeatherBENCH unifies the field by providing a benchmark dataset that has been tailored for machine learning models, as well as presenting an evaluation metric that various models can be compared with.
Hopefully, by standardizing these elements of the machine learning process in weather forecasting, WeatherBENCH can be the groundwork for vastly improved forecasting models.
References
- Ackerman, Frank, and Elizabeth A. Stanton. “The Cost of Climate Change.” NRDC.org, National Resource Defense Council, 2008, www.nrdc.org/sites/default/files/cost.pdf.
- Rasp, Stephan, et al. “WEATHERBENCH: A BENCHMARK DATASET FOR DATA-DRIVEN WEATHER FORECASTING.” Climatechange.ai, Climate Change AI, 2020, www.climatechange.ai/papers/iclr2020/11/paper.pdf.
- Taylor, Matthew, and Jonathan Watts. “Revealed: the 20 Firms behind a Third of All Carbon Emissions.” The Guardian, Guardian News and Media, 9 Oct. 2019, www.theguardian.com/environment/2019/oct/09/revealed-20-firms-third-carbon-emissions.
- M. Hayati, Z. Mohebi, et al. “Deep Learning-Based Effective Fine-Grained Weather Forecasting Model.” Pattern Analysis and Applications, Springer London, 2020, link.springer.com/article/10.1007/s10044-020-00898-1.